Let me start by saying that no part of this article was written by ChatGPT. While I’m confident that it would have been a beautifully articulate piece, generated in seconds, it would not have been me.
By now most of you have heard about the new Generative AI capability that has taken the world by storm. ChatGPT is the fastest growing application in history with one million users in the first five days of release and 100 million users in two months. In January of 2023, it had recorded thirteen million unique visitors per day, doubling from the previous month. After years of hype, the promise of practical artificial intelligence has become a reality. People all over the world are leveraging this technology to write legal documents, draw up contracts, craft press releases, write code, and even create touching letters to those they love. The application of ChatGPT seems to be endless.
Many technologies have had the promise of making our lives easier, but none have come anywhere close to the impact that ChatGPT and other Generative AI applications will have. While many of these advancements, like the Internet and social media, have had a major impact on the world, none have ever been embraced as quickly, or have caused as much excitement, fear, and disruption in such a short period of time. The world has found the ‘easy’ button through Generative AI, and the implications are staggering.
Let’s consider how it could affect our daily lives. Could it impact songwriters, filmmakers, and authors by generating content with differences that are almost imperceptible by the audience? Could it challenge legal structures for trademarks, IP, patents, and copyrights by generating voluminous content through a single question? Could it force teachers and professors to question the validity of every paper they receive? Could it make phishing and dark-web-based attacks more frequent and even more potent? Could it replace people and impact jobs?
The answer to these questions and thousands more like them is yes! Generative AI is a fundamental change in the way that humans will operate. It’s as if eight billion people received a personal assistant with unlimited capabilities and knowledge overnight.

What is Generative AI, GPT, and ChatGPT?
Like any new technology, understanding how to use it can seem daunting. The beauty of ChatGPT is they’ve removed the complexity. If you can ask a question, you can use the technology. I have two objectives for this article. The first is to help readers understand what happens under the hood. The second is to ensure that the digital infrastructure industry is fully aware of the implications of the adoption of this technology.
McKinsey defines Generative AI as an artificial intelligence that describes algorithms, such as ChatGPT, that can be used to create new content, including audio, code, images, text, simulations, and videos.
Tech Target defines GPT (Generative Pre-Trained Transformer) as a machine learning model trained, using Internet data, to generate any type of text.
OpenAI defines ChatGPT as a model they trained that interacts in a conversational way. Essentially it is a chat application that leverages the GPT model.
What is magical about ChatGPT is that it can create compelling content at blinding speed. When you input a small amount of text, usually in the form of a question, it will generate large volumes of relevant and sophisticated machine-generated text. The big question is how does ChatGPT do this? That comes down to how the models are trained. GPT is now in its fourth generation. GPT4 is by far the most powerful large language model ever built. Let me put this into perspective through table 1.
Launch | Model Parameters | Growth | |||
GPT1 | June, 2018 | 117,000,000 | 117M | – | – |
GPT2 | February, 2019 | 1,500,000,000 | 1.5B | 13x | 9 Months |
GPT3 | June, 2020 | 175,000,000,000 | 175B | 117x | 16 months |
GPT4 | March, 2023 | 100,000,000,000,000 | 100T | 571x | 33 months |
The parameters that are used to train the GPT model have grown exponentially. As you can see in the chart, each generation has a huge increase in training parameters compared to the previous version. It has grown 854,700 times its original number in less than five years – mind blowing.
In 2021 I published an article in the eighth edition of Interglobix Magazine titled “Defining the Digital Infrastructure Industry.” The goal was to create a common definition for digital infrastructure to help establish a starting point for global capacity and consumption. This taxonomy allowed us to measure growth, efficiency, and the carbon impact of the digital infrastructure that serves people and machines.
In 2021 there were seven million data centers with 105GW of capacity that consuming 594TWhs of energy, which represented 2.4 percent of global energy consumption. Looking back, there were predictions that the amount of global energy consumed by digital infrastructure would reach double digits. Those predictions did not materialize due to a number of factors. First were the breakthroughs in compute performance that allowed us to grow the number of transactions while slowing, flattening, and even, in some cases, decreasing the watts required to complete that work. Second was the focus on data center facilities to remove waste by adopting a metric called PUE—Power Usage Effectiveness. While workload demand increased, the amount of capacity needed to power, cool, and ensure that the data centers stayed up, declined. The result was a continuous doubling of transactions per watt of power consumed.
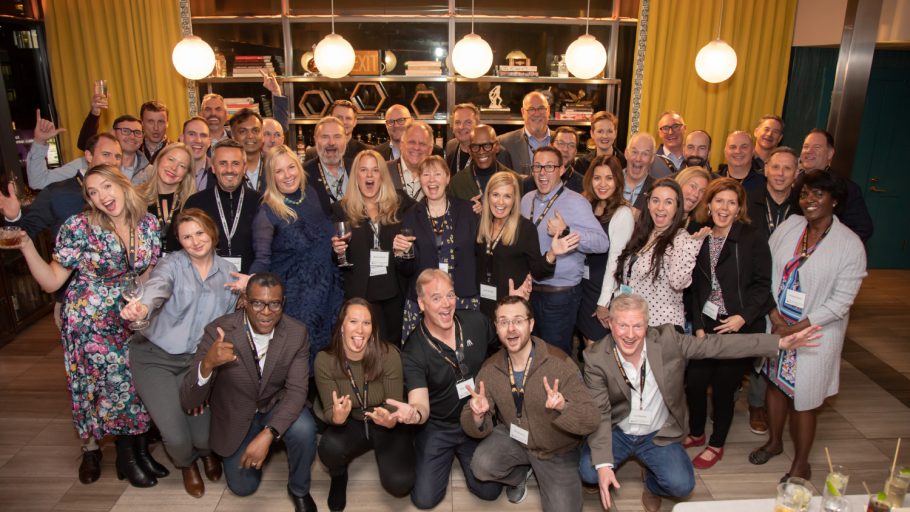
I’ve been in this industry for over 30 years. Every year there has been hype about the next technology that will double demand, drive density, and increase utilization upward for every rack in the data center. While there has been an increase, it has not been a hockey stick. The growth has been gradual. Over the last twenty years, rack densities have gone from an average between 2–6kW/rack to 8–15kW/rack. While this represents a 2.5–4x growth, it is nowhere near the predicted averages exceeding 20kW+/rack. Don’t get me wrong, there are many 25kW, 50kW, and even 100kW+ rack deployments, but they represent less than ten percent of the total capacity used in data centers. True high density is a niche market for workloads like high-performance computing, search, and of course, machine learning and AI applications.
The Impact
What has happened in digital infrastructure over the past three decades will pale in comparison to what is coming because of Generative AI. ChatGPT is the fastest growing application in history. While that is impressive, ChatGPT is only one application of Generative AI from one company. First, demand will increase faster than at any other time in our history. Second, we are unable to deliver on that demand as the technology is deployed today. Training GPT4 models on a global scale is not economically or ecologically viable. Consider table 2.
Launch | Model Parameter Growth | Power Consumed to train model | Metric Tons of CO2 | Equivalent homes powered for a year | ||
GPT1 | June, 2018 | 117M | 0 | – | – | – |
GPT2 | February, 2019 | 1.5B | 12.8x | – | – | – |
GPT3 | June, 2020 | 175B | 117x | 1,287 MWh | 552 | 100 |
GPT4 | March, 2023 | 100T | 571x | 4,166 MWh | 1,787 | 324 |
Mosharaf Chowdhury, an associate professor at The University of Michigan, published a report showing that it took 1,287MWh to fully train GPT3. That is the same as powering 100+ US homes for a year.
Kasper Groes Albin Ludvigsen, a Danish data scientist, used this data to extrapolate how much power will be required to train the GPT4 model. He correlated the consumption from BLOOM, a similar model with 176 billion parameters, with the work of Dylan Patel and Afzal Ahmad at SemiAnalysis to validate the prediction. Kasper believes it takes 4,166MWh to train GPT4 fully. That is the same as powering 324 US homes for a year.
The main thing to consider when analyzing this table is the amount of power needed to train the specific generation of GPT. On one hand, the parameters used to train the GPT4 model increased 571 times, but the power consumption only increased 3.2 times. That’s a good thing as each generation of GPT is more efficient, even with the explosive growth of parameters.
On the other hand, the GPT4 model will not be trained just once. It will be trained over and over again in both public and private settings.
Public Use
GPT4 is based on 100 trillion parameters that have been collected across the Internet. OpenAI has a version of ChatGPT via a web browser or mobile browser on a smartphone. Microsoft, via its OpenAI investment, has also embedded GPT4 into Bing and is incorporating it into Office 365 applications. Microsoft’s deployments are very good for users, as GPT4 has more current information included in the model (GPT4 has info up to 2023 versus GPT3 which has info up to November 2021), and it is now citing references. The bad side is that, as time passes and more data comes in, the GPT4 model(s) must be trained again.
Private Use
One of the biggest risks to corporations, governments, military organizations, and other entities is exposure of data when using ChatGPT publicly. While there are safeguards defined for the use of ChatGPT, it is very new, and most people are not considering the implications. For example, using ChatGPT to write code or providing input data like personal data or copyrighted / IP data into ChatGPT has inherent risks. Because of these concerns, some companies and countries have banned the use of ChatGPT. In contrast, other companies are now deploying their own internal Chat v GPT-like services to mitigate these risks.
Retraining
Whether ChatGPT is used publicly or privately, the models must be retrained over time. What this means is each time the full GPT4 model has to be retrained, the company doing the training will consume another 4,166MWh and emit another 1,787 metric tons of CO2.
What to expect
With the adoption of ChatGPT and other Generative AI applications, there will be a tsunami of data creation, which will force the models to be retrained again and again. What does this mean to digital infrastructure capacity and consumption? Could our industry’s 2.4 percent energy draw double? Triple? Potentially go into double digits? This question is open, but in my opinion we are facing unprecedented demand, which will translate to significant capacity requirements. Make no mistake: companies are focused on creating the easy button for people and machines to do more, faster. This means the world will continue to generate data faster than at every other time in history.
What gives me hope is every advancement has counterbalances that act as forcing functions. In this case, those balancing factors are cost and public commitments.

Money Drives Behavior
Power consumption for these models will be balanced by cost. Companies don’t have unlimited budgets. Cost pressures are a forcing function to drive efficiency, advancements in performance, and acceptable tradeoffs. For example, incremental learning may evolve to help Generative AI reduce the number of times the models are retrained or updated, which would lower the power consumption and the cost.
Public Commitments Drive Behavior
Carbon emissions will be reduced through companies’ public ESG commitments. This includes new growth, such as the demands from Generative AI. The biggest companies in the world, including Microsoft, Meta, Google, and AWS, have committed to aggressive carbon reduction goals. That includes uniting on industry efforts like the iMasons Climate Accord to compound the results across digital infrastructure. Like economic costs, companies cannot afford to increase carbon emissions and still meet their aggressive reduction goals. This forcing function will drive innovation, which will drive new cost-effective, decarbonized solutions.
All disruptive technologies, like the steam engine, the printing press, the Internet, and Generative AI, drive change in business models, economics, and of course, human behavior. These changes can be both good and bad. Those who try to stop their advancement are fighting the wrong battle. In my opinion, Generative AI presents an equally compelling advancement and concern in the way humans operate. The rules are changing. The pace is accelerating. The implications are massive. We need to learn to use the technology to our advantage while balancing out the impact. From a digital infrastructure perspective, we need to plan for the growth that will be driven by Generative AI adoption, but we cannot approach it in the same manner. To achieve economic and ecological balance, we need to rethink our approach and operate with scarcity in mind. We must do more with less. As Christian Belady has reminded me time and time again, the best innovations happen during times of constraint. We’re definitely constrained on multiple levels. It’s time to get creative, folks!